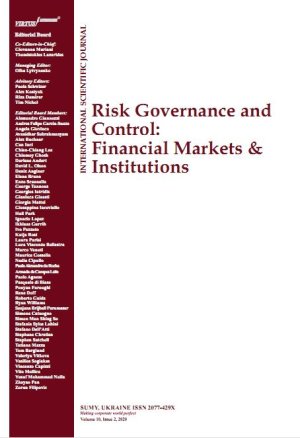
A COMPARATIVE STUDY OF STOCK PRICE FORECASTING USING NONLINEAR MODELS
Download This Article
This work is licensed under a Creative Commons Attribution-NonCommercial 4.0 International License.
Abstract
This study compared the in-sample forecasting accuracy of three forecasting nonlinear models namely: the Smooth Transition Regression (STR) model, the Threshold Autoregressive (TAR) model and the Markov-switching Autoregressive (MS-AR) model. Nonlinearity tests were used to confirm the validity of the assumptions of the study. The study used model selection criteria, SBC to select the optimal lag order and for the selection of appropriate models. The Mean Square Error (MSE), Mean Absolute Error (MAE) and Root Mean Square Error (RMSE) served as the error measures in evaluating the forecasting ability of the models. The MS-AR models proved to perform well with lower error measures as compared to LSTR and TAR models in most cases.
Keywords: Stock Price, Nonlinear Time Series Models, Error Metrics
Received: 01.08.2016
Accepted: 12.02.2017
How to cite this paper: Xaba, D., Moroke, N.D., Arkaah, J. and Pooe, C. (2017). A Comparative Study Of Stock Price Forecasting Using Nonlinear Models. Risk governance & control: financial markets & institutions, 7(2), 7-17. https://doi.org/10.22495/rgcv7i2art1