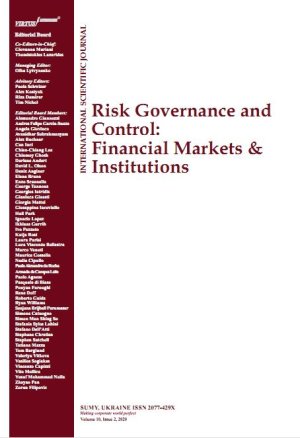
Machine learning in accounting: Insight from the March 2023 bank failures
Download This Article
This work is licensed under a Creative Commons Attribution 4.0 International License.
Abstract
This research investigates the bank failures in the United States in March 2023, concentrating on the impact of held-to-maturity debt instruments in the event and the implications for accounting methods. Our research deciphers the alleged “accounting loophole” (Farrell, 2023) associated with these securities and provides an in-depth analysis of the associated accounting treatment. We analyze the accounting treatment using the Accounting Standards Codification (ASC) and International Financial Reporting Standards (IFRS). Furthermore, our study employs automated machine learning techniques and the local interpretable model-agnostic explanations (LIME) method to identify key accounting features that could explain bank failures. The research identifies five essential accounting aspects, two of which are related to held-to-maturity assets. The findings underscore the importance of these accounting features in evaluating financial institutions, thereby providing valuable insights for stakeholders, decision-makers, and future research. Our research also advocates for increased transparency and accuracy in accounting practices, via ASC 825 (Financial Accounting Standards Board [FASB], n.d.-a), particularly related to the fair value of held-to-maturity securities.
Keywords: Held-to-Maturity, Bank Failure, Content Analysis, Automated Machine Learning, Financial Statements, Local Interpretable Model-Agnostic Explanations (LIME)
Authors’ individual contribution: Conceptualization — M.M. and Y.A.; Methodology — M.M.; Formal Analysis — M.M. and Y.A.; Writing — M.M. and Y.A.; Project Administration — M.M. and Y.A.
Declaration of conflicting interests: The Authors declare that there is no conflict of interest.
JEL Classification: G21, G33, M40, M41, M49
Received: 19.03.2023
Accepted: 31.05.2023
Published online: 02.06.2023
How to cite this paper: Mulyadi, M., & Anwar, Y. (2023). Machine learning in accounting: Insight from the March 2023 bank failures. Risk Governance and Control: Financial Markets & Institutions, 13(2), 28–36. https://doi.org/10.22495/rgcv13i2p3