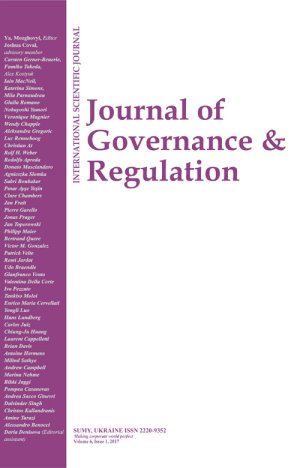
Predictive analytics in customer behavior: Unveiling economic and governance insights through machine learning
Download This Article
This work is licensed under a Creative Commons Attribution 4.0 International License.
Abstract
This study addresses the challenge of predicting customer behavior in the Thai e-commerce sector using machine learning (ML) models. The research aims to evaluate the effectiveness of random forest and gradient boosting models, benchmarking them against traditional models such as logistic regression and linear regression. Utilizing a dataset from a leading Thai e-commerce platform, the study identifies key predictors of customer satisfaction and spending power, including purchase frequency, age, and customer referrals. The findings reveal that ML models outperform traditional methods in capturing complex, non-linear relationships within consumer data. These results suggest practical applications in customer segmentation, personalized marketing, and optimized resource allocation (Chen et al., 2012). The study also emphasizes the importance of ethical considerations, such as transparency and data privacy, in predictive analytics. The research contributes to the integration of technology and economics, offering insights into the potential of ML in understanding and influencing consumer behavior (Wamba et al., 2015).
Keywords: Predictive Analytics, Machine Learning Models, Customer Behavior, E-Commerce, Consumer Data Privacy
Authors’ individual contribution: Conceptualization — R.K. and N.Y.; Methodology — R.K. and N.Y.; Software — R.K.; Validation — R.K., T.K., and N.Y.; Formal Analysis — R.K. and T.K.; Investigation — R.K., T.K., and N.Y.; Resources — R.K. and T.K.; Writing — R.K. and T.K.; Supervision — N.Y.
Declaration of conflicting interests: The Authors declare that there is no conflict of interest.
JEL Classification: C53, C55, D12, M31, L81
Received: 08.05.2024
Revised: 16.08.2024; 29.01.2025
Accepted: 14.02.2025
Published online: 18.02.2025
How to cite this paper: Kasemrat, R., Kraiwanit, T., & Yuenyong, N. (2025). Predictive analytics in customer behavior: Unveiling economic and governance insights through machine learning [Special issue]. Journal of Governance & Regulation, 14(1), 318–331. https://doi.org/10.22495/jgrv14i1siart8