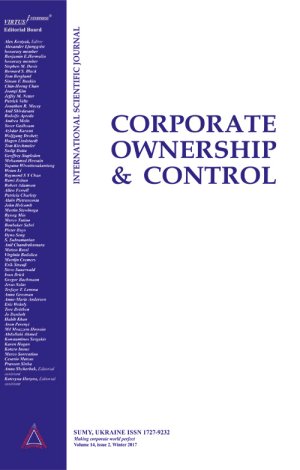
-
Journal menu
- General information
- Editorial Board and External Reviewers
- Journal Policies
- Publication Ethics and Malpractice Statement
- Instructions for authors
- Paper reviewing
- Article processing charge
- Feedback from stakeholders
- Journal’s Open Access statement
- Order hard copies of the journal
- 50 most cited papers in the journal
- Statement on the Use of Generative AI
Decision information for auditors to assess litigation risk: Application of machine learning techniques
Download This Article
This work is licensed under a Creative Commons Attribution 4.0 International License.
Abstract
Fraud cases have become more common in recent years, highlighting the role of auditors’ legal liability. The competent authorities have called for stricter control and disciplinary measures for auditors, increasing auditors’ legal liability and litigation risk. This study used machine learning (ML) techniques to construct a litigation warning model for auditors to assess audit risk when they evaluate whether accept or terminate an engagement, thus improving audit quality and preventing losses due to litigation. Otherwise, a sample matching method comprised of 64 litigated companies and 128 non-litigated companies was used in this study. First, feature selection technology was used to extract six important influencing factors among the many variables affecting auditors’ litigation risk. Then a decision tree was used to establish a litigation warning model and a decision table for auditors’ reference. The results indicated that the eight outcomes provided by the decision table could effectively distinguish the level of a litigation risk with an accuracy rate of 92.708%. These results can provide useful information to aid auditors in assessing engagement decisions.
Keywords: Litigation Risk, Machine Learning, Feature Selection, Classifier Technique
Authors’ individual contribution: Conceptualization — Y.-H.L.; Methodology — Y.-H.L.; Formal Analysis — Y.-C.L.; Resources — F.-C.G.; Writing — Y.-H.L. and Y.-C.L.; Supervision — Y.-H.L.
Declaration of conflicting interests: The Authors declare that there is no conflict of interest.
JEL Classification: M4, C8, M1
Received: 15.03.2022
Accepted: 06.05.2022
Published online: 10.05.2022
How to cite this paper: Lu, Y.-H., Lin, Y.-C., & Gu, F.-C. (2022). Decision information for auditors to assess litigation risk: Application of machine learning techniques. Corporate Ownership & Control, 19(3), 133–146. https://doi.org/10.22495/cocv19i3art10