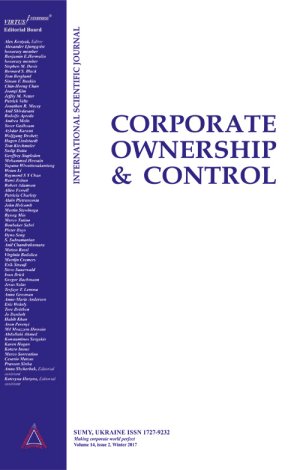
-
Journal menu
- General information
- Editorial Board and External Reviewers
- Journal Policies
- Publication Ethics and Malpractice Statement
- Instructions for authors
- Paper reviewing
- Article processing charge
- Feedback from stakeholders
- Journal’s Open Access statement
- Order hard copies of the journal
- 50 most cited papers in the journal
IMPROVED ALGORITHM FOR CLEANING HIGH FREQUENCY DATA: AN ANALYSIS OF FOREIGN CURRENCY
Download This ArticleAbstract
High-frequency data are notorious for their noise and asynchrony, which may bias or contaminate the empirical analysis of prices and returns. In this study, we develop a novel data filtering approach that simultaneously addresses volatility clustering and irregular spacing, which are inherent characteristics of high-frequency data. Using high frequency currency data collected at five-minute intervals, we find the presence of vast microstructure noise coupled with random volatility clusters, and observe an extremely non-Gaussian distribution of returns. To process non-Gaussian high-frequency data for time series modelling, we propose two efficient and robust standardisation methods that cater for volatility clusters, which clean the data and achieve near-normal distributions. We show that the filtering process efficiently cleans high-frequency data for use in empirical settings while retaining the underlying distributional properties.
Keywords: High Frequency, Market Microstructure, Realized Volatility, Volatility Clusters
How to cite this paper: Jayawardena, N. I., West, J., Li, B., & Todorova, N.(2015). Improved algorithm for cleaning high frequency data: An analysis of foreign currency. Corporate Ownership & Control, 12(3-1), 125-132. https://doi.org/10.22495/cocv12i3c1p1